Dear reader,
During my first two years in San Francisco, I tried hard to shut out singularity talk. I avoided AI doomers and anyone who self-described as “building the machine god.” For a community professedly interested in protecting humanity against extinction, the rhetoric of AGI seemed immature and willfully obtuse. Believers couch their work in mysticism, repeating vague mantras like “What did Ilya see?” and “Feel the AGI.”
But one by one, more folks I know started converting. One friend visited SF to figure out whether to quit his finance job for a frontier lab. On a walk around Alamo Square, he mused aloud: “Will AI actually be revolutionary, or just a normal technology like the internet or phones?” Wow, I thought. The internet and phones seemed like big deals to me! And LLMs and Waymos already feel like magic—every time ChatGPT identifies a bird photo or a car turns its own wheel, I’m struck with a small surge of unguarded awe.
So I decided to start wrestling with these ideas head-on to understand their grip. I began asking friends for introductions to the “most AGI-pilled person you know” and assembling a syllabus of seminal forum posts and research papers. Using LLMs daily has been helpful too—feeling out the edges of what they can and can’t do.
I wouldn’t call myself a believer yet, though I’ve updated in the direction that yes, AI really matters. And it’s therefore worrying that for a technology so transformative, analysis around how AI will fit into our human world remains rudimentary. Will we tax the labs? How should education change? Is Xi Jinping an AI doomer? I keep asking and learning that none of these questions have good answers yet. Meanwhile, many of the strongest thinkers about society and politics—the people I want most guiding diffusion—continue to believe that LLMs are buggy auto-complete.1
Some of the stakes of AI are technical—which I’ll explicate when relevant—but equally consequential is the worldview of the insular communities building it. The entire way we talk about AI—the lingo, the timelines, the risk scenarios that dominate policy conversations—is downstream of 50 people in a hyper-niche subculture of (mostly) Bay Area transhumanists, rationalists, and effective altruists. Just as we are living out the consequences of Mark Zuckerberg’s belief in instant global connection and Steve Jobs’ “bicycle for the mind,” we will soon be subject to the world that Sam Altman (or Dario, or Elon, or Demis) prophesied. I want to uncover what that world is.
what is AGI?
I’m starting with a simple-sounding question: What is AGI?
The leading labs have all informally named building AGI as their explicit goal, yet there’s surprisingly little consensus on what it means. News articles do this hand-wavey trick where they spell out “artificial general intelligence,” then wedge a definition like “AI better than humans at cognitive tasks” between two em-dashes before moving on. But I’ve never found that very elucidating. Better than some humans, or all of them? What counts as a ‘cognitive’ task—doing your taxes, writing a poem, negotiating a salary, falling in love?
I brought my questions to a friend, who shrugged it off as semantic. “We’ll know AGI when we see it,” he said. “It’s like that court case about obscenity.” I asked an AI engineer I knew the same question, but he replied that answering was impossible: “It’s like asking when aliens will land on earth.” I’m surprised at their confidence in porn and aliens, to be honest; if we still can’t settle disputes about nipples and UFOs, how do we expect to agree on the nature of intelligence itself?
This sent me down a rabbit-hole. My friend is right, it’s semantic, but these words are lenses to understand what researchers are devoting their lives (and billions) to build. They reflect changing technological capabilities, commercial pressures, and philosophical assumptions about intelligence itself. What follows is an abbreviated history of how visions of AGI have evolved—and retreated—over the past ~75 years, from the academic fathers of computing to today’s frontier labs. (If you want a timeline sans commentary, I made one here.)
Finally, I conclude that AGI is more a worldview than technology—nobody knows when we’ll get there, or where “there” is—and we may be better off focusing on the AIs we build along the way.
a short semantic history of AI
Since antiquity, humans have fantasized about creating machines as capable and intelligent as we are. Of course, the earliest robots were mechanical, and possessed no cognitive skill.
By the mid-20th century, the first electronic computers were performing complex calculations and cracking the ciphers that helped the Allies win World War II. These feats expanded scientists’ imaginations, putting “thinking” machines within reach. In 1947, Alan Turing first proposed the idea of computing machines with “general” capabilities that could “do any job that could be done by a human computer in one ten-thousandth of the time.” In 1955, John McCarthy kicked off the Dartmouth Summer Research Project on AI to study the simulation of human intelligence; covering topics like reasoning, language, and recursive self-improvement.
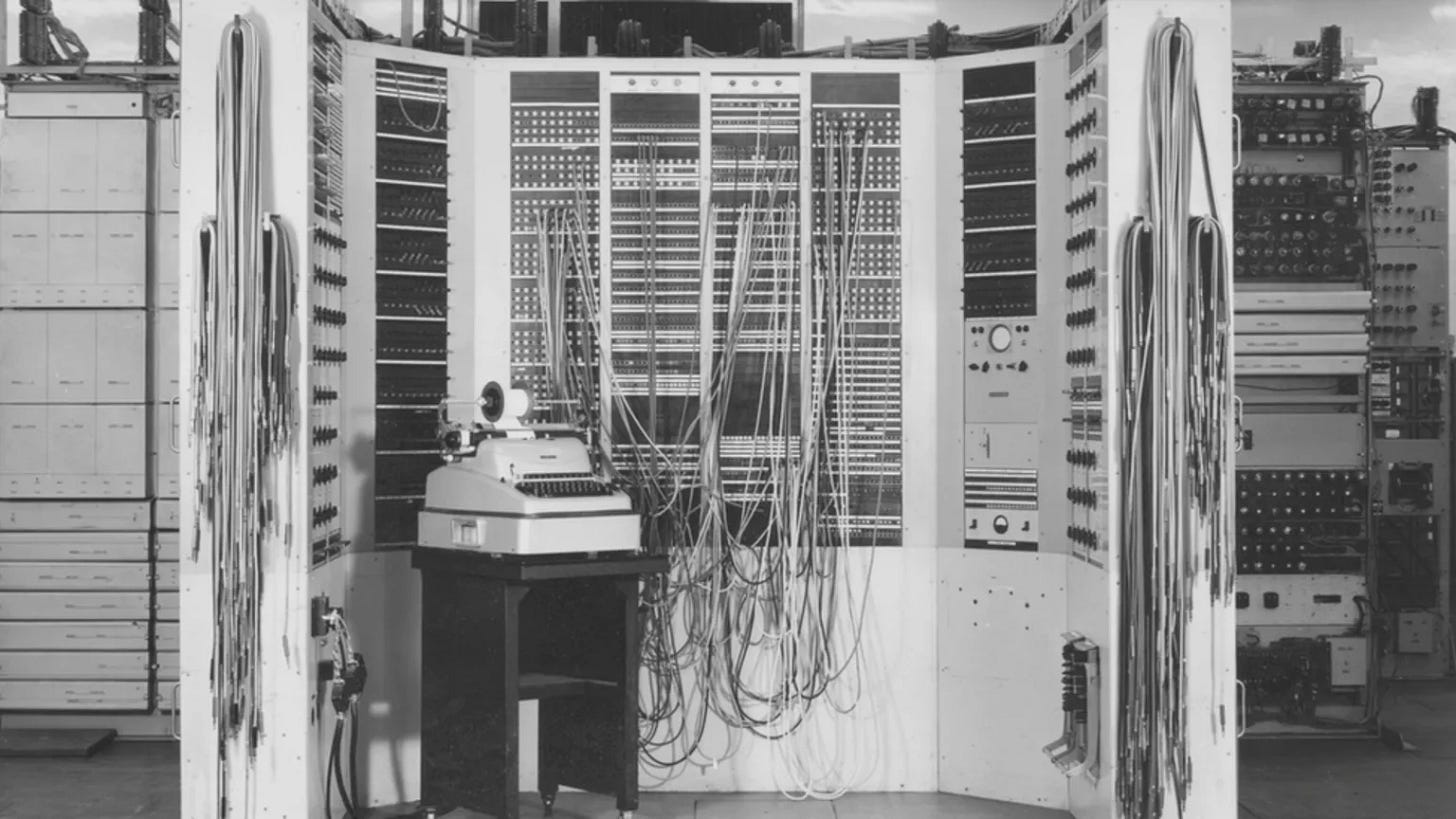
For the next several decades, early AI pioneers stumbled through formal logic, hand-coded rules, and expert systems; trying and failing to get their brittle systems to live up to their lofty visions. It was only when scientists like Geoff Hinton and Judea Pearl introduced statistical methods in the 1980s—training models to learn from data, not follow pre-written formal rules—that AI started to sort-of work. While Bayesian networks and backpropagation were major breakthroughs, the AI systems they enabled were “narrow,” meaning they could only perform the single task they were trained for. Backgammon-playing computers were amazing, but far from Turing’s original dreams.
The early 2000s revived “generality” as a serious goal. The term AGI was coined and popularized in 2002 by Ben Goertzel and Shane Legg (now DeepMind’s cofounder and “Chief AGI Scientist”). Goertzel wanted a term to title his book, organize a budding intellectual community, and distinguish it from research on narrow AI. Goertzel writes:
What is meant by AGI is, loosely speaking, AI systems that possess a reasonable degree of self-understanding and autonomous self-control, and have the ability to solve a variety of complex problems in a variety of contexts, and to learn to solve new problems that they didn’t know about at the time of their creation.
This is an ambitious threshold. It’s not just about problem-solving, but also metacognition (self-understanding, autonomy) and the ability to adapt to novel environments. In the years after publishing the book, Goertzel would convene the inaugural Conference on AGI in Bethesda, and AGI gained momentum in online forums and futurist listservs. In a 2011 paper, Nick Bostrom and Eliezer Yudkowsky endorsed AGI as “the emerging term of art used to denote ‘real’ AI.”
But like many grand missions, AGI’s meaning would be forced to evolve alongside its community. For the next two decades, researchers would continue to debate, reinterpret, replace, and reformulate the term, all the while working toward the technology’s invention.
Shane Legg and Mark Hutter, in 2008, characterized “intelligence” as “an agent’s ability to achieve goals in a wide range of environments,” then attempted a formal mathematical definition of “universal intelligence.” Notably, they found metacognitive criteria too narrow—machines might not exhibit the specific mental processes that humans do.
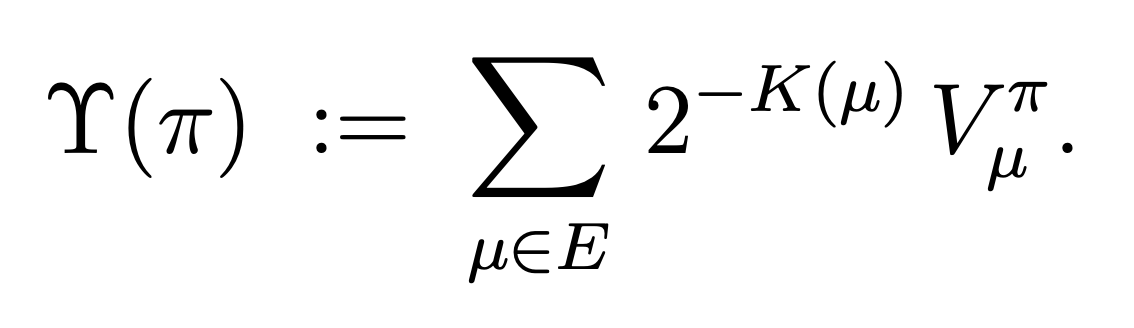
Others preferred “human-level AI” or “human-level machine intelligence” (often abbreviated as HLMI). Adopters included AI pioneers like McCarthy and Nils Nilsson. The latter proposed an “employment test” to replace the Turing Test:
AI programs must be able to perform the jobs ordinarily performed by humans. Progress toward human-level AI could then be measured by the fraction of these jobs that can be acceptably performed by machines.
HLMI carries the advantage of being neutral, grokkable, and pragmatic. Like Legg and Hutter’s definition, it’s a functional standard—an AI is advanced because of what it can do, not how it works. Bostrom uses HLMI instead of AGI in his surveys of AI experts,2 as does Katja Grace. HLMI also admits its anthropocentrism: per Yann LeCun, “Even human intelligence is very specialized.” And there’s good reason that human benchmarking has held water—it’s a clear proxy for learning, generalization, economic impact, and the like.3
Holden Karnofsky argues that the most important AI milestone is broad societal impact, not what the AI looks like or how it works. His effective altruist nonprofit, Open Philanthropy, is chiefly interested in mitigating the risks of “transformative AI.”
A potential future AI that precipitates a transition comparable to (or more significant than) the agricultural or industrial revolution. [...] “Transformative AI” is intended to [leave] open the possibility of AI systems that count as “transformative” despite lacking many abilities humans have.
“AGI” in the LLMs era
Nevertheless, AGI has persisted as most insiders’ term of choice. The term is sticky, even as its specifications shift.
DeepMind released a “Levels of AGI” paper in 2024, outlining how both narrow and general models might progress past tiers of human skill. The authors suggest that most modern understandings of AGI correspond to the “Competent AGI” cell—just one level deeper than the current frontier. Autonomy, cognition, and real-world impacts, they argue, are not required for AGI.
Across the Atlantic, Anthropic CEO Dario Amodei eschews AGI—too “religious” and “sci-fi”—for “powerful AI” and “Expert-Level Science and Engineering.” The latter is especially revealing—Anthropic aims to win the race by first automating AI research itself, hence why Claude excels at coding.
OpenAI also employs a practical definition of AGI in their mission: “to ensure AGI—highly autonomous systems that outperform humans at most economically valuable work—benefits all of humanity.” The company’s agreement with Microsoft demands greater specificity. Per The Information, their contract stipulates AGI as when OpenAI develops AI systems that can reach $100 billion in profits (~$20b less than Saudi Aramco made in 2024, but ~$3b more than Apple).4
This hard-nosed financial interpretation is amusing but unsurprising. Taking a birds’ eye view of the last half-century of AGI discourse, definitions have inched toward the measurable, practical, and achievable; emphasizing an AI’s performance—especially vis-a-vis human benchmarks, on human jobs—over internal “consciousness” or “agency,” recursive self-improvement, or the ability to generalize to any new task or environment.
Some argue that this reflects the industry’s broader shift toward applications and commercialization; as
and quip, “AI companies are pivoting from creating gods to building products.” Others complain that AGI definitions are being watered down or overfitted to current models to obscure the slowing rate of LLM progress: Altman’s latest formulation (“a system that can tackle increasingly complex problems, at human level, in many fields”) seems particularly weak.There are holdouts, of course. Francois Chollet continues to defend generality as the critical precondition for AGI, conceptualizing “the intelligence of a system [as] a measure of its skill-acquisition efficiency over a scope of tasks, with respect to priors, experience, and generalization difficulty.” He is uninterested in automating ordinary tasks, which he argues can be achieved through mere memorization; his ARC-AGI benchmarks require models to solve strange visual puzzles not found in their training data (Chollet manually designs them to be easy for humans but hard for computers). While I’m philosophically sympathetic, I’m not sure his test is any better. Most jobs are more than bundles of tasks; they require agency, relational skills, and adapting to change.
AGI as a social construct
After poring through a century of varied conceptualizations, I’ll write out my current stance, half-baked as it is:
I think “AGI” is better understood through the lenses of faith, field-building, and ingroup signaling than as a concrete technical milestone. AGI represents an ambition and an aspiration; a Schelling point, a shibboleth.
The AGI-pilled share the belief that we will soon build machines more cognitively capable than ourselves—that humans won’t retain our species hegemony on intelligence for long.5 Many AGI researchers view their project as something like raising a genius alien child: We have an obligation to be the best parents we can, instilling the model with knowledge and moral guidance, yet understanding the limits of our understanding and control.6 The specific milestones aren’t important: it’s a feeling of existential weight.
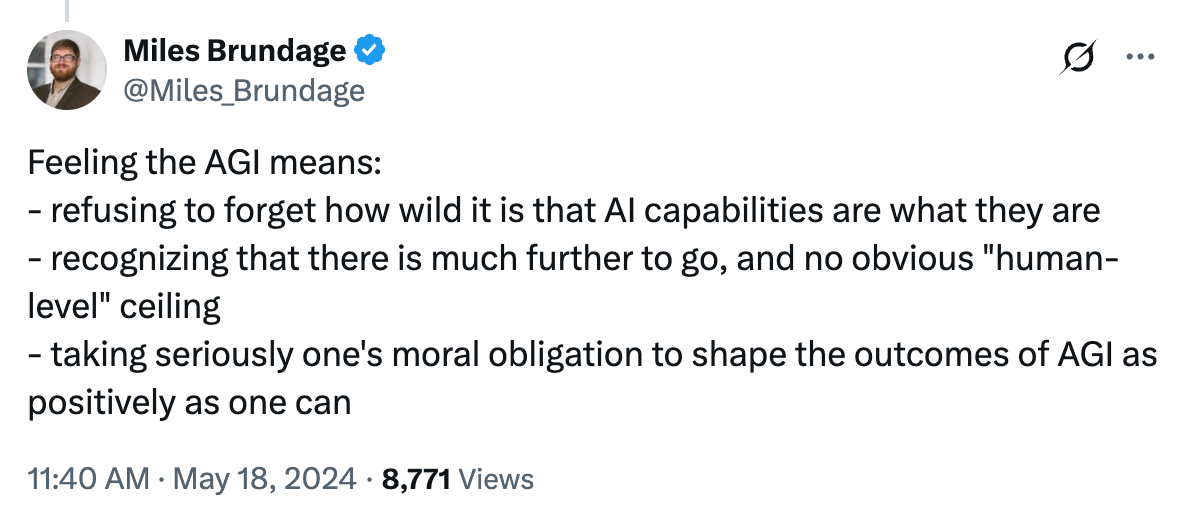
However, the definition debates suggest that we won’t know AGI when we see it. Instead, it’ll play out more like this: Some company will declare that it reached AGI first, maybe an upstart trying to make a splash or raise a round, maybe after acing a slate of benchmarks. We’ll all argue on Twitter over whether it counts, and the argument will be fiercer if the model is internal-only and/or not open-weights. Regulators will take a second look. Enterprise software will be sold. All the while, the outside world will look basically the same as the day before.7
I’d like to accept this anti-climactic outcome sooner than later. Decades of contention will not be resolved next year. AGI is not like nuclear weapons, where you either have it or you don’t; even electricity took decades to diffuse. Current LLMs have already surpassed the first two levels on OpenAI and DeepMind’s progress ladders. A(G)I does matter, but it will arrive—no, is already arriving—in fits and starts.8
Climate science debates offer an instructive analogy. Since Malcolm Gladwell published The Tipping Point at the turn of the millennium, “tipping points” scenarios have dominated climate discourse. The metaphor suggests that there are specific critical thresholds (popularly 1.5 degrees warming) after which runaway climate change will destroy the planet and lead humanity to extinction. Supposedly these are points of no return, after which self-reinforcing cycles in melting ice sheets and decaying rainforests will accelerate impacts even if emissions stop.
But contrary to popular understanding, there are multiple regional climate tipping points, and scientists cannot predict exactly what they are. Our environment is incomprehensibly complex, yet tipping points rhetoric encourages a reductive “Are we there yet?” mentality that ping-pongs people between complacency and doom. Meanwhile, climate impacts like wildfires and species loss advance unevenly but surely across the earth. As policymakers quibble over the precise date the apocalypse will hit, the planet dies a slow death beneath our feet.
I suspect the story of AI may look quite similar. Harmful incidents—security breaches, misinformation, job loss, deception, discrimination, misuse—are happening today. So are AI’s benefits, like human augmentation and accessibility. These impacts will get magnified as AI systems become more powerful and autonomous, whether or not AGI has been formally declared. And like with climate change, we ought to frame AI progress not as a binary of safe/unsafe or AGI/not-AGI, but as a jagged frontier of capabilities with risks that depend on where you’re looking.9 AI discovered wholly new proteins before it could count the ‘r’s in ‘strawberry’, which makes it neither vaporware nor a demigod but a secret third thing.
This model of AI development is significantly more boring and hard to describe, and I will probably keep saying “building AGI” because it’s a good shorthand for a particular outlook. But I maintain that an all-or-nothing view of AGI is misleading and counterproductive: humans have agency to govern and adapt to AI as it improves and diffuses, in different forms in different contexts at different levels; and our current mitigations will lay the groundwork for long-term risks too. This is not an argument for passivity or against forecasting; to the contrary, it’s an appeal to start paying attention now. Rather than an unbroken arc of innovation hurtling straight from Deep Blue to Skynet, the dialectical dance between technological progress and societal response may save us after all.
misc links & thoughts
I’m still stumbling my way into fluency around AI stuff, so very open to corrections, additions, critiques, etc. There’s probably a bunch that’s wrong here—I am nervous to publish this because of how novice I feel, but figured that I’ll learn faster by failing in public.
I need longer than the scope of this essay to understand the FOOM stuff, but it currently seems improbable to me. The thing about our markets and institutions is that extremely smart, powerful, resourced individuals are still constrained from taking over and killing everyone else. And the thing about AI research is that it isn’t just raw coding ability, but also creativity, collaboration, time, and physical resources. These bottlenecks seem very real, and we will increase those constraints as the first serious AI impacts start to occur (e.g. a Three Mile Island scenario). But this is all pretty weakly held, and at some point I’ll read everything and talk to more people and see if I change my mind.
Other AI questions on my mind: Why are so many of the technical titans of AI also the most concerned about alignment and safety? Where are the good policy ideas for adapting to economic displacement, if it happens? What do the biggest power users or AI-natives’ lives look like (especially outside of tech)? What do I think the biggest ~risks~ and ~opportunities~ are?
I’m 3 months into Substacking/podcasting/freelancing now! I’d love feedback from subscribers on what you’d like to see more of—both form (e.g. essay, podcast, reporting, book review) and topic area (e.g. tech, politics, personal, som hyper-specific explainer…). I know you’re supposed to just write what you like, but I enjoy most things so any feedback helps a lot—just hit reply.
Thanks for reading. Tell me your timelines!
Jasmine
Many tech critics have become so terrified of “critihype” that they’ve abdicated their actual job, which is to anticipate and examine how frontier tech may change society in ways good and bad. And yes, that requires some speculation about things that haven’t happened yet. Hype-busting is useful but cannot be the whole game!
Bostrom spells out HLMI as “high-level machine intelligence,” but defines it AI “that can carry out most human professions at least as well as a typical human,” so I’m using it interchangeably with the other HLMI.
For Bostrom, HLMI is just a milestone en route to superintelligence: what happens after AGI is achieved, when machines “greatly exceed the cognitive performance of humans in virtually all domains of interest.” That world is when he thinks the greatest risks will emerge.
On the
in 2023, Shane Legg explained why he moved toward human-level benchmarking for AGI: it’s the most natural “reference machine” for any measure of a task’s complexity.Many speculate that the “AGI clause” incentivizes OpenAI to declare AGI sooner, since it revokes Microsoft’s right to use its technologies.
In this metaphor, “alignment” and “‘interpretability” are hard for the reasons that human parenting is hard. There is a risk that—perhaps through no fault of your own—your child will be both brilliant and utterly amoral, ultimately turning on the very beings that ushered it into the world.
I wonder what the incentives are for a company to “declare AGI.” For OpenAI, it’ll get them out of their Microsoft agreement, and perhaps it’d help a startup generate recruiting/fundraising hype; but whoever does it would invite a ton of scrutiny and backlash—and it could even usher in a micro-winter if “AGI” doesn’t meet expectations. I almost wonder if a Chinese lab might go for it first.
Sam Altman, Fei-Fei Li, etc. have said as much.
DeepMind’s “Levels of AGI” paper says something along these lines in Section 6.1 and Table 2 at the end, though their primary consideration there is levels of autonomy rather than generality or performance.
For another picture of how this might play out, a recent paper by Atoosa Kasirzadeh outlines the “accumulative AI x-risk hypothesis,” embracing a complex systems view of AI:
Accumulative AI x-risk results from the build-up of a series of smaller, lower-severity AI-induced disruptions over time, collectively and gradually weakening systemic resilience until a triggering event causes unrecoverable collapse.
Jasmine, I have so many thoughts about this, I almost feel compelled to blog about it. Probably the thing that annoys me most is the "skeptics" crowd is so underrepresented in this debate. I'm an AGI skeptic and disagree with almost everything Arvind, Sayash, and Francois write.
For now, I wanted to share my AGI reading list that I made in 2019. Despite the half decade of impressive chatbot results, all of them hold up remarkably well and explain the *mindset* of your AGI bro on the street.
Ted Chiang's critique of the threat of superintelligence.
https://www.buzzfeednews.com/article/tedchiang/the-real-danger-to-civilization-isnt-ai-its-runaway
Maciej Cegłowski's Superintelligence: The Idea That Eats Smart People.
https://idlewords.com/talks/superintelligence.htm
Stephanie Dick’s history of our conceptions of artificial vs natural intelligence.
https://hdsr.mitpress.mit.edu/pub/0aytgrau/release/3
David Leslie's scathing review of Stuart Russell's book.
https://www.nature.com/articles/d41586-019-02939-0
Thanks for breaking this down. I probably need one more level of translation simply because I’m illiterate in much of this.
I appreciate your understanding of regional climate tipping points. I think you are asking :: does the date really matter? Don’t we know in our bones that we need to figure out how to live on the planet without harming it? If so, amen. Thank you.
I hope you are enjoying your life on the outside of this epic platform.
Thanks for all you did on the inside 🌱🌿💚